How Predictive Analytics can Influence Employee Engagement?
Need to know:
- Most employers are already harvesting ample data to engage in some form of predicative analytics, from sources such as engagement surveys and benefits take up statistics.
- Regression analysis can help track potential exit drivers and problem areas for engagement, while predicative models can map how initiatives will affect factors such as retention.
- All data must be treated with care, however, to remain compliant and ensure top quality predictions, and the human touch is still integral.
Data analysis has revolutionized the consumer experience, and as employers shift away from seeing stats as mere cogs in the machine, it is set to do the same for the workplace.
Although only 11% currently use advanced techniques, 50% of employers think predicative analytics would help gauge employee sentiment and improve engagement, according to research by MHR Analytics, published in June 2019.
Doug Brown, chief data scientist at Capita, says: “Most organizations are going through something of a change and transformation. [With data analysis] they’ve got an ability to understand what their organization is culturally, or what they want to move to, and what the [drivers] are that they can influence.
“That might be pay, fi, work conditions, a whole set of things that drive employees to be happy at work, improving overall productivity.”
Data sources
The type of information being harvested will vary according to what an employer is trying to achieve.
Volume can be key, says Brad Winsor, vice president of workforce analytics at business analytics firm SplashBI. “The whole point is to capture the breadth and height of the employee experience. [Breadth] means every attribute [organisations] know about the employee, and then the height is over time.”
Information is often already at an organisation’s fingertips, says Brown. “Employers have a lot of data they have harvested and stored, but [have] not necessarily always collated and aggregated,” he explains. “It’s a question of how do [employers] aggregate that data, and then how can they focus that into insight?”
Sources might include employee demographic data, usage information held on benefits systems and recognition platforms, payroll, time and attendance statistics, engagement and pulse surveys, gender pay gap data, and external measures such as Glassdoor reviews.
Evolving uses
Many organisations will already be using some form of analytics to map the effectiveness of their benefits packages, says Nick Shaw, chief executive offi cer of workplace psychology firm 10x Psychology. “What was the spend, and did people take them up? That’s fairly straightforward. Then, there are different data points people can connect that to: did that mean they stayed in their job for longer? [Would they] recommend the [organisation]?”
Regression analysis, in which a programme looks back at a sample of data, such as an employee’s activities and attributes over a six-month period, correlating that with another factor, such as whether they stayed with the organisation, can help employers recognise patterns.
Once an employer has the factors, it can tweak them and change the data fed into the regression analysis, and consider, ‘what if we’d done this in the past, what would have happened?’ says Winsor.
In this way, employers can gain a deeper understanding of how certain initiatives will likely impact retention and engagement across the business.
Laura Timms, product manager at MHR Analytics, says: “It might just pull out things [employers have] never really thought about, or identify patterns [they have] never really got to before, automating processes, saving time and also backing up decisions with the evidence [needed] to get buy-in from the business.”
Employee wellbeing
Just as marking out potential exit drivers can flag up when to consider how to better engage certain members of staff, spotti ng warning signs for physical or mental health deterioration can help direct staff to the relevant interventions.
According to Innovation generation: The big HR tech disconnect 2019/20, published by Thomsons Online Benefits in July 2019, 33% of employers already collect staff health data from wearable devices; by 2020, 83% plan to analyse information such as footfall and desk time in order to influence wellbeing strategy.
“Whether it’s about sleep or health or eating patterns, [employers can] direct people towards initiatives and interventions that will actually help them and that are relevant,” says Shaw. “Organisations will be challenged to think more about diagnosis, so that the interventions they put in place are meaningful and impactful.”
The limitaons of data
Although the potential benefits are manifold, any use of data comes with warnings, not least from a compliance perspective, following the introduction of the General Data Protection Regulations (GDPR) in 2018.
“[Employers] have to start with a clear user need, benefit and ethical purpose around different sets of data,” says Brown. “[There] should be a net good to society, delivering value to all parties, with an expectation of tangible benefit.”
In addition, although data analysis can combat unconscious bias by spotti ng patterns uninfluenced by personal assumptions, this is only true if the information being fed in, for example relating to progression or hiring patterns, has not itself been affected by historical bias.
Decision support
Whether linking intangible concepts such as engagement with hard statistics, or providing insights into patterns that might not have been spotted by the human eye, data analysis can be the helping hand in shoring up decision-making, targeting initiatives and undoing bias.
At least for now, though, the human touch is still important. Shaw concludes: “Sometimes people just want the silver bullet on a decision, but there isn’t such a thing, and the data isn’t always right. There’s a lot to be said for using the insights, but [also] using common sense and judgement, not just taking the data as gospel.”
Related Posts
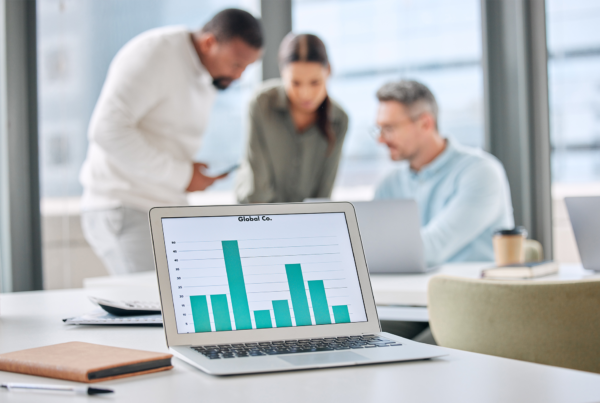
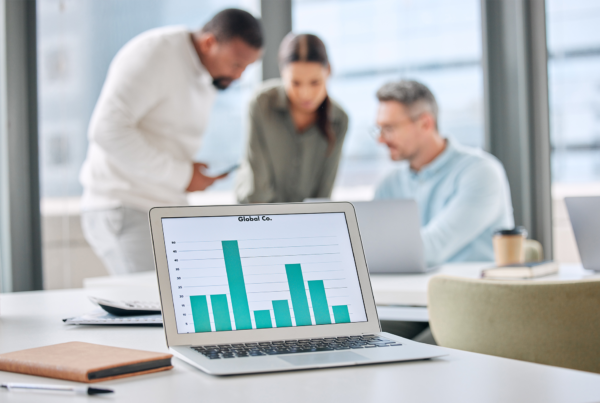
Comprehensive Payroll Reporting with SplashBI for Fusion HCM
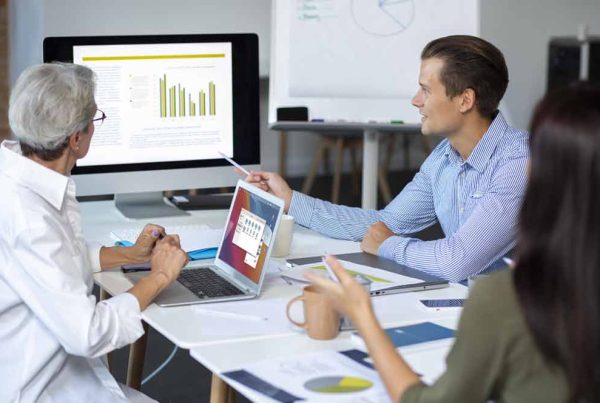
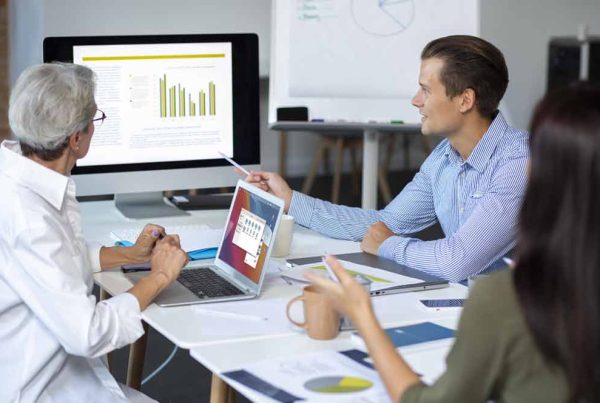
5 Must-Have Strategies to Close the Gender Pay Gap with People Analytics
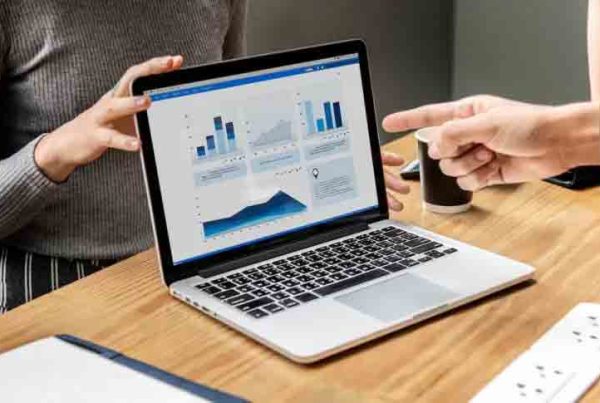
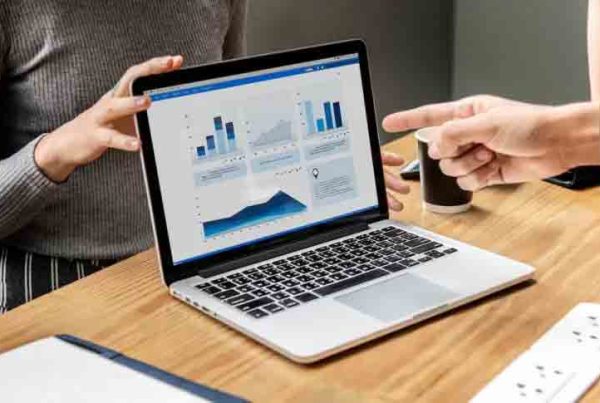