Leverage Anomaly Detection to Elevate People Analytics Insights
Introduction
Data analytics mainly focuses on finding patterns or study the KPIs that influence the business. However, there is one classification of data that is discarded in the process-outliers.
Outliers are considered as unexpected deviations in the standard patterns but can help identify anomalies. Anomaly detection is a prominent field in data-driven studies and can uncover growth opportunities or expose an area of concern.
Be it fraud detection in financial transactions or health monitoring through alterations in ECG scans; it has many practical applications in the business. This article gives an overview of anomaly detection and discusses few potential areas in HR where anomaly detection techniques can prove helpful.
What is Anomaly Detection?
By definition, anomaly detection identifies events or observations that do not conform to an expected pattern or other items in the dataset. Often, the term anomaly detection and outlier detection is used interchangeably.
The anomalies can highlight any spike (positive or negative) by measuring the deviations from the normal baseline. An example of a negative spike can be sudden deterioration in employee performance compared to previous years. On the other hand, an increase in training undertaken by an employee is a positive spike & presents an opportunity to move the employee to a more suitable role. An anomaly can be analyzed as an isolated incident or a cluster of outliers (collective & contextual anomalies) like, very low employee engagement in a group of employees.
Data is the new information currency of the organizations. The data is extensively analyzed to derive business insights in all the areas. Customarily, data analytics focuses on innovative methods to highlight patterns and relationships. They are derived using the historical data gathered over time. The collected data is modeled through various machine-learning algorithms and provides the baseline for key performance indicators (KPIs). During this process, the outliers are often discarded to facilitate data modeling. These outliers, if studied, have the potential to give valuable business insights.
Regardless of the KPIs contained in the collected data, anomaly detection is all about finding and fixing incidents as they are happening. Anomaly detection helps uncover the unknown from the unknowns & assists in capitalizing on hidden opportunities or addresses incidents proactively.
Dedicated Anomaly Detection provides the following value addition to the Analytics:
1. Real-time analysis:
Employ anomaly detection feature to incorporate real-time alerts. The criticality of the event decides the frequency of anomaly detection. For example, an anomaly detector should immediately notify managers if a female employee leaves the office after hours to promote employee safety. Working late hours can cause employee dissatisfaction and result in attrition in female employees. In the absence of anomaly detection, these incidents surface later, leading to missing opportunities.
2. Dynamic monitoring:
Rather than setting manual thresholds, we can use machine-learning algorithms to create a dynamic baseline. A metric’s behavior can fluctuate due to seasonality, industry standards, or geographic location, making it prudent to set up thresholds dynamically. An anomaly detection algorithm continuously analyzes the business data to understand its normal behavior under different conditions and seasons, thus preventing false alerts.
3. Granular Insights:
The traditional dashboards provide a holistic view of the business metrics. The focus is on conventional data silos and fails to highlight isolated & significant anomalies. The event stands out only when it affects business metrics. The inclusion of anomaly detection provides insights at the granular level, which may otherwise go unnoticed. The recurrence of anomalies may highlight a brewing situation and can be used to cut time to remediation.
Anomaly Detection pertinent to People Analytics
Anomaly detection imparts new capabilities to the analytics space. While it elevates the analytic solution, it is equally important to do it right. It is especially challenging in the HR domain, as the HR ecosystem usually collects data from different sources. Spotting anomalies over varying systems is highly complex because it is difficult to establish relationships across data sources. This is where intelligent feature engineering & domain expertise comes into play. The domain expert is responsible for identifying relevant features related to potential pain areas based on experience and historical data. A central data repository with only the required features can mitigate the complexity and facilitate outlier detection.
Having established the importance of anomaly detection, we discuss its applicability in People Analytics. In HR management, it can be used to expose the possibility of burnout in employees. During COVID-19, the ‘work-from-home’ culture has led to longer working hours, no vacations/leaves, working on weekends. This has resulted in fatigue in the employees, thereby affecting productivity or developing health issues. Anomaly detection can highlight these potential problem areas, compelling the manager to give compulsory time-off to the employee. Apart from this, identifying the possibility of fatigue can also prevent accidents & injuries at the workplace.
Anomaly detection can vastly improve payroll-related activities in an organization. Common anomalies include duplicate employee details, employees with no tax filing details, an employee with no bank details, employee pay rates not matching their award rate, and a few more.
Further, identifying a location where the number of payroll runs is much higher than the average value can uncover the need for payroll training. An employee hitting any tax or deduction with a limit set by the business would typically show up as an anomaly.
Anomaly detection at the gross-to-net calculation level flags any significant deviations from last month’s data. These anomalies are specific to aspects of payroll or other HR-related business processes. For payroll, moving the anomaly detection up in the processing cycle can elevate the quality of payroll processing. Applying an algorithm to identify anomalies to inputs received via integration & resolve before running the payroll is one such opportunity. This will reduce the number of off-cycle payroll runs and improve the employee experience by ensuring employees are paid timely & correctly.
Similarly, the processes related to outbound interfaces present another improvement opportunity. For example, anomaly detection can be applied to ensure that a tax file consistent with the last transmission is sent out. Deviations can be manually checked to determine their correctness. This gives additional assurance to payroll teams and can prevent hefty penalties or interest in case of incorrectness.
Conclusion
These are some of the ideas where People analytics can take advantage of advanced analytics and anomaly detection to better view their data. Integrating HR with financials, CRM, infrastructure, etc., can facilitate cross-metric correlation to uncover more granular insights.
Want to learn more about People Analytics or want to engage in a meaningful conversation around the subject? We are SplashBI for HR!
Related Posts
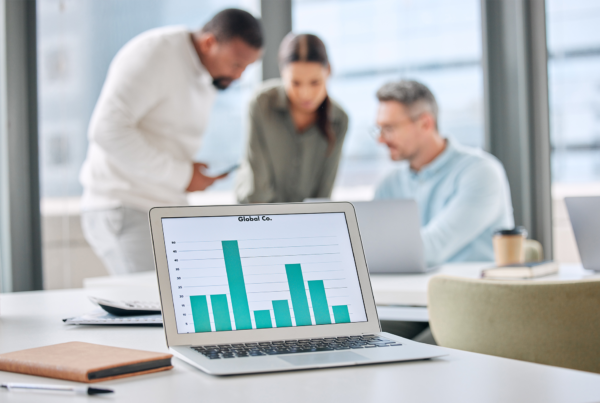
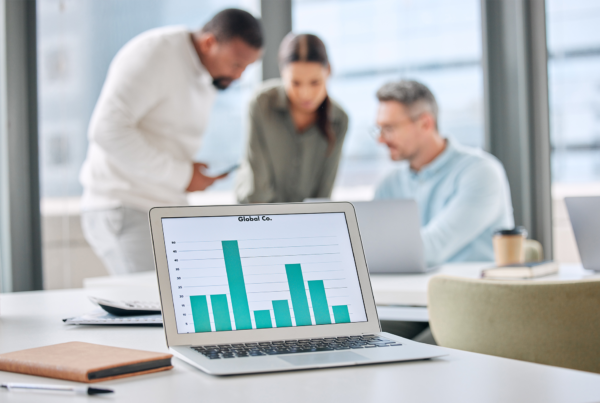
Comprehensive Payroll Reporting with SplashBI for Fusion HCM
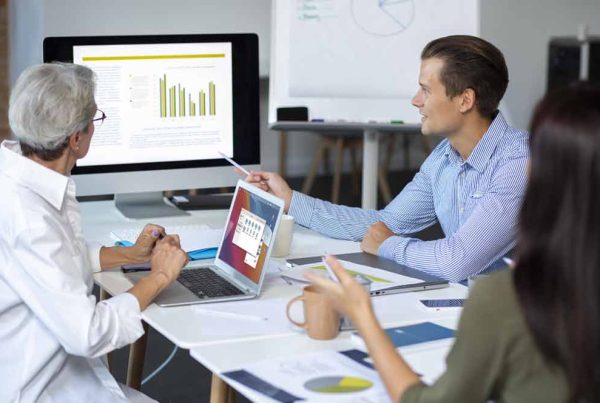
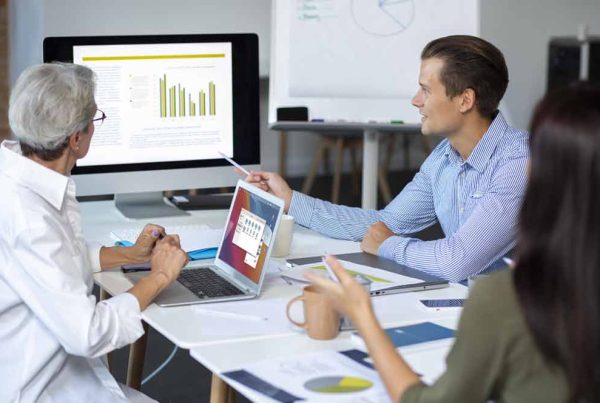
5 Must-Have Strategies to Close the Gender Pay Gap with People Analytics
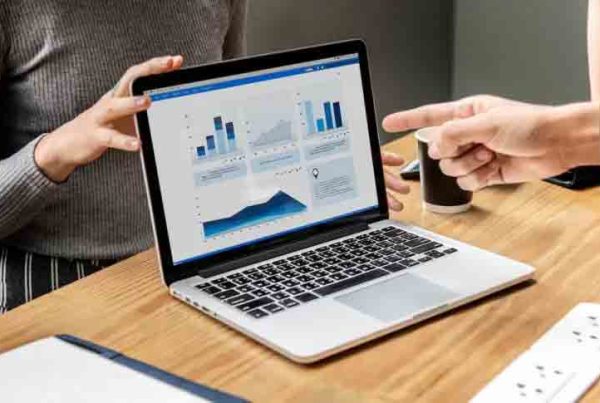
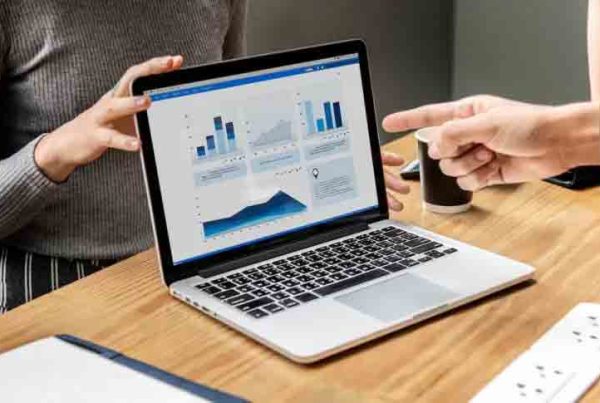